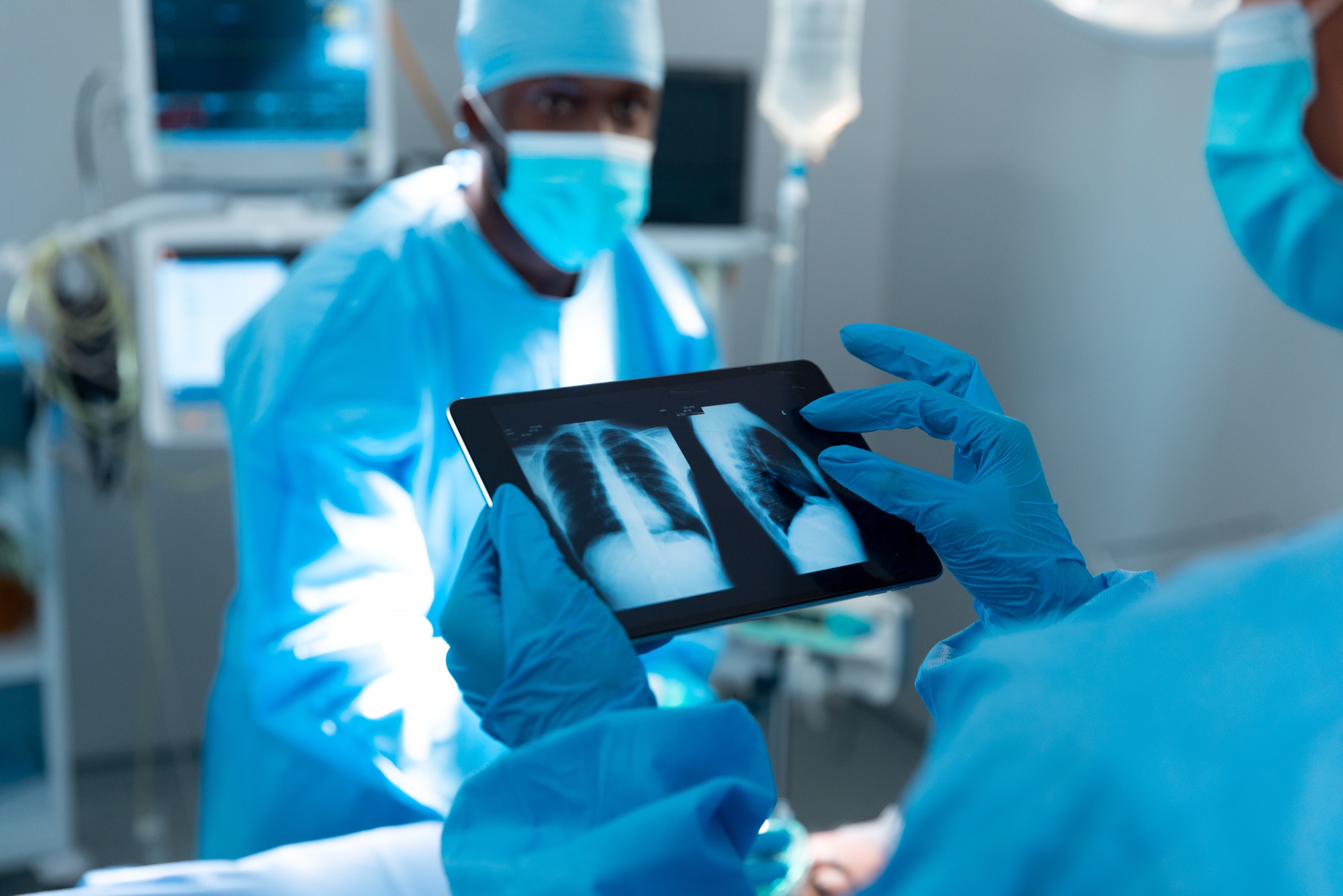
AI for Health - Covid Detection
Improve the workflow of radiologists by automating lung segmentation in thorax X-rays to detect Covid
Premium participation: Get 1-on-1 Mentoring & Guidance
Try a free mentoring sessionDetecting Covid in patients with X-rays
Challenge completed. Watch the recording of the AI for Health - Covid Detection final results presentations to discover that the teams came up with:
About the Challenge
Thorax (chest) X-rays are one of the most common medical imaging techniques used to diagnose lung disease. At the beginning of the Corona pandemic, this was one of the most trusted techniques to diagnose Covid in hospitalized patients. To be sure everyone infected with Covid would be appointed to the right hospital wing all newly hospitalized patients went through an X-ray screening.
This took up a tremendous amount of time for radiologists. A robust AI model would make a great impact if it would be capable of automated Covid detection. To train such an algorithm, it’s important that the model only picks up true signs of Covid and ignores the “noise”. Noise is all the unwanted features in an image and other forms of data that cause the model to overfit. To reduce noise in the dataset the images needed to be segmented.
Info session
Our challenge partner
GOAL: Automatic segmentation of lungs using Generative Adversarial Models
The first step of the challenge will be aimed at doing research on GANs and image generation. After that we will start building our own GAN. We’ll screen the currently available segmented data if it’s suited for the training. We’ll experiment with building various loss functions. The quality goal we’ll try to reach will be model accuracy of over 90%.
Who are we looking for?
We are looking for 5 experienced AI engineers and data scientists available as soon as possible since we will be addressing an extremely time critical subject.
Timeline
Application Deadline: 18 April 2021
Final Presentations: 18 June 2021