Advancing preservation of biodiversity with the help of AI
10
partners
5+
Challenges per year
250+
AI for Wildlife Engineers
25,000+
hrs of Engineering
Multiplying AI Engineering Skills for Wildlife Protection
Labs by FruitPunch AI build a community of AI engineers with an ecosystem of partners around a specific topic and technology. AI for Wildlife Lab bundles the experience from all challenges into resources for machine learning projects zooming in on biodiversity protection. It helps its members and partners to strengthen their network with other organizations operating in the niche.
A personal quest to protect wildlife
In the very beginning of the non-profit initiative, to become later FruitPunch AI, Buster Franken was looking for a way to make an impact in the real world. Buster put out a call to action to apply AI to solve problems related to the UN Sustainable Development Goals. The wildlife reserves of South Africa responded and weeks later, Buster was in the bush in Pilanesberg, SA to see how AI could help in their fight against poaching. He witnessed the trail of destruction left by poachers.
He knew he couldn’t do this alone so he recruited co-founder Sako Arts and 50 AI engineers from around the globe to tackle the beast. For 10 weeks, they worked closely together with the rangers to develop an autonomous drone with thermal cameras to detect poachers. This was the first AI for Good Challenge - AI for Wildlife.
Wildlife has a special place in our hearts and its conservation is an important part of the the UN Sustainable Goal #15 - Life of Land. With the AI for Wildlife Lab we’ll be able to do much more to protect it.
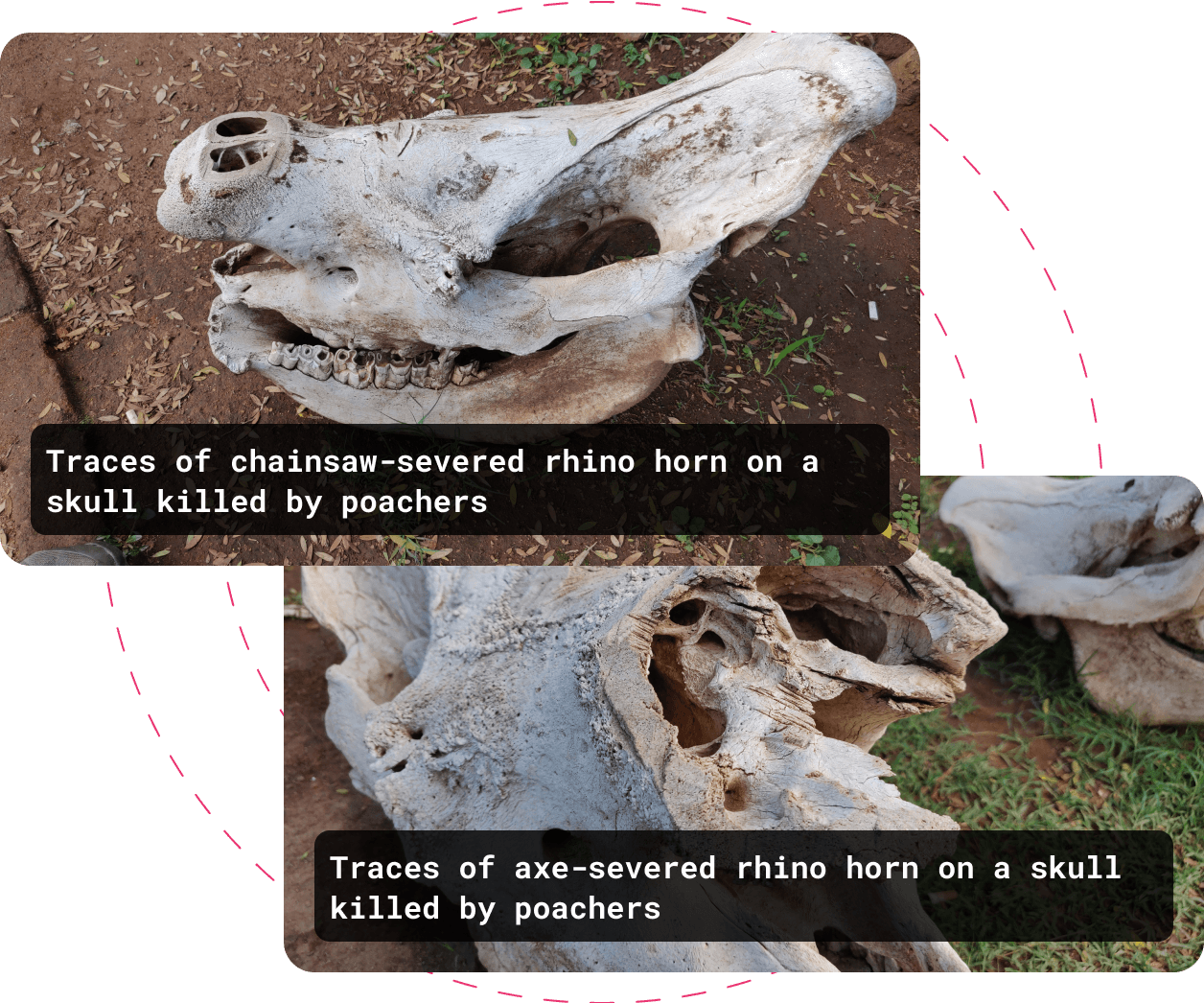
UN Sustainable Development Goal 15: Life on Land
Protect, restore and promote sustainable use of terrestrial ecosystems, sustainably manage forests, combat desertification, and halt and reverse land degradation and halt biodiversity loss.
12
Targets
15
Events
31
Publications
908
Actions
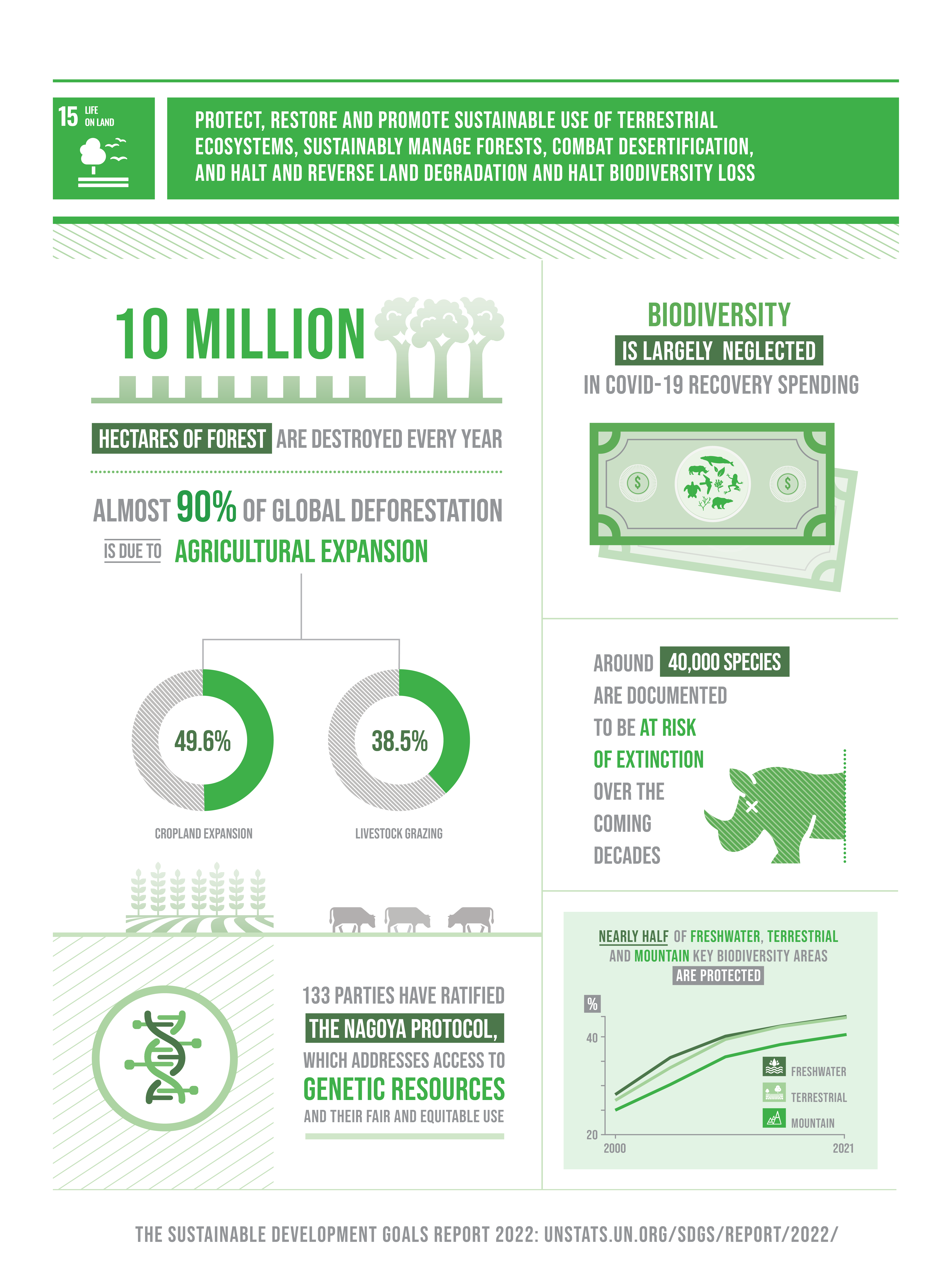
Build a community of skilled AI for Wildlife Engineers using machine learning to tackle wildlife conservation
Organize as many successful AI for Wildlife Challenges as possible
Build a network of partners active in the wildlife preservation niche and bundle their resources
Get the most out of the combined engineering effort by focusing on edge computing, computer vision and tiny ML technology
Edge Computing
When doing wildlife conservation in remote areas, any machine learning faces a lack of connectivity. You can’t connect to a server to compute object detection from the bush. The computer vision model has to run on the available hardware on edge. Edge computing in machine learning translates into energy-efficient models that are able to do more work independently, without being constantly plugged into the cloud.

Tiny ML
Closely tied to edge computing, tiny ML approaches try to create model miniatures that are not draining batteries or burning one GPUs. It’s very important for a battery-powered poacher-detecting drone flying over South African reserves with patchy connectivity. It tackles some fundamental challenges to machine learning - energy consumption, latency, storage and data security.

Computer Vision
Computer vision is a common use case in machine learning, but applying perception models on edge requires next level AI skills. Our AI for Wildlife engineers have to implement a working MLOps to improve poacher detection and figure out how to coexist with other edge MLs (like autonomous flight functions) under fairly tricky conditions.
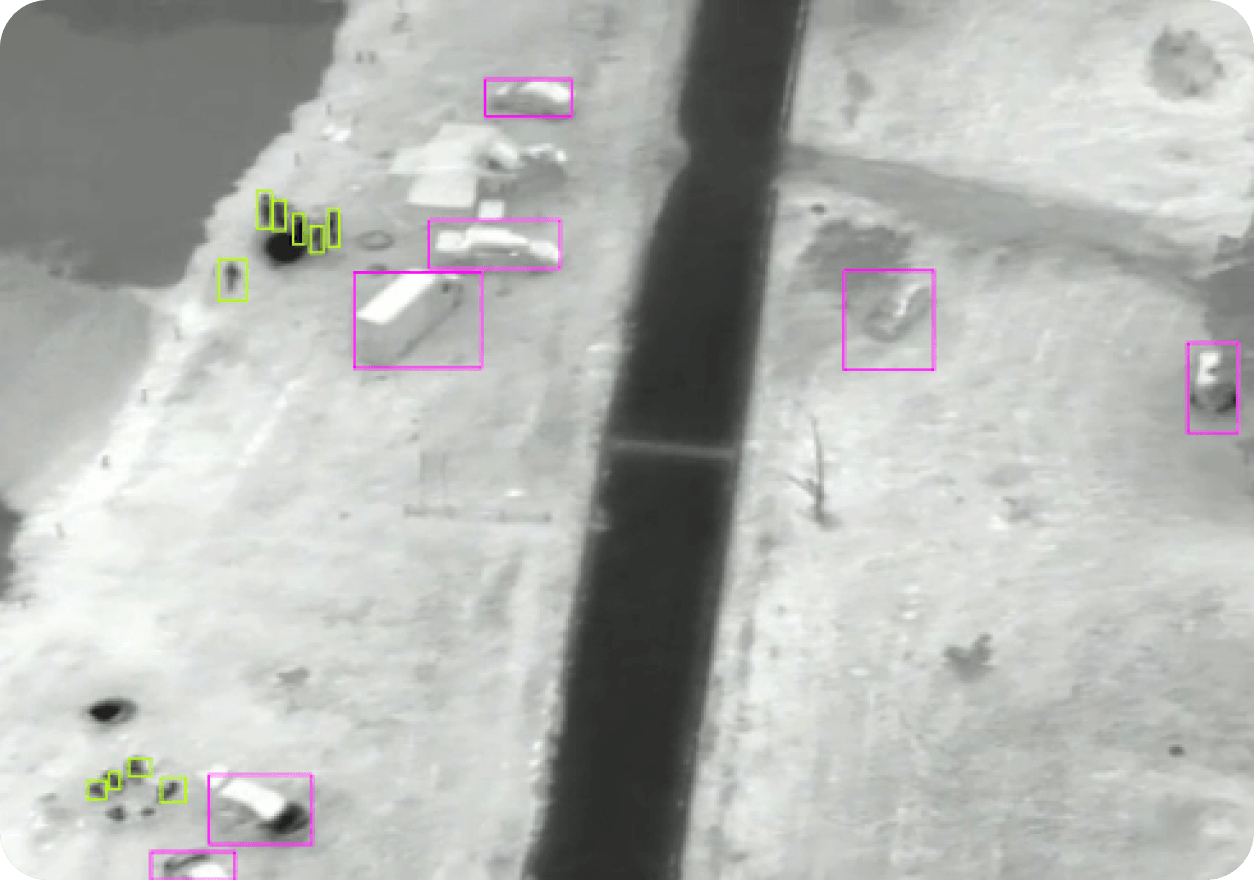
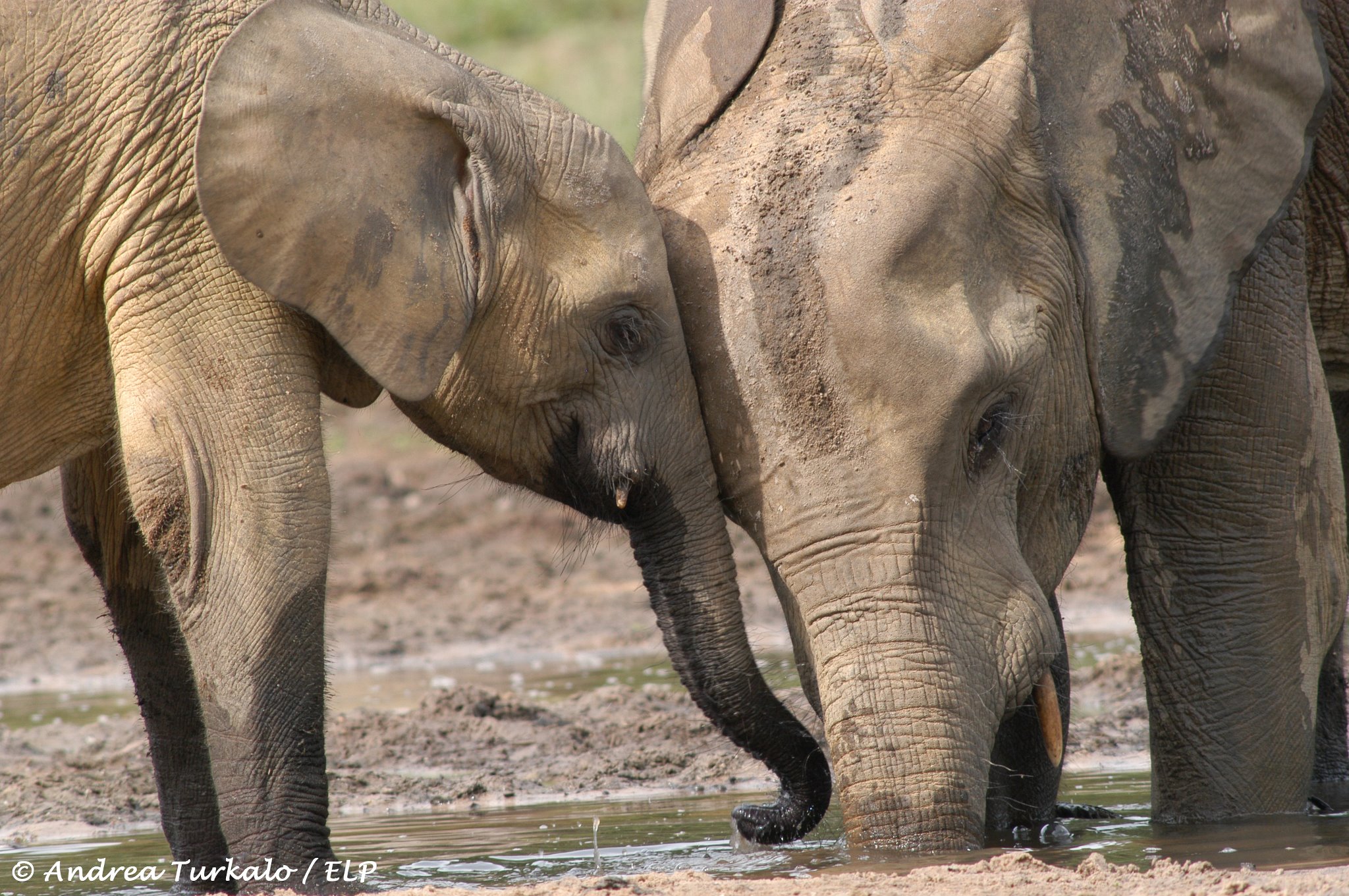
AI for Forest Elephants 2

AI for Bears

AI for Coral Reefs 2

AI for Turtles
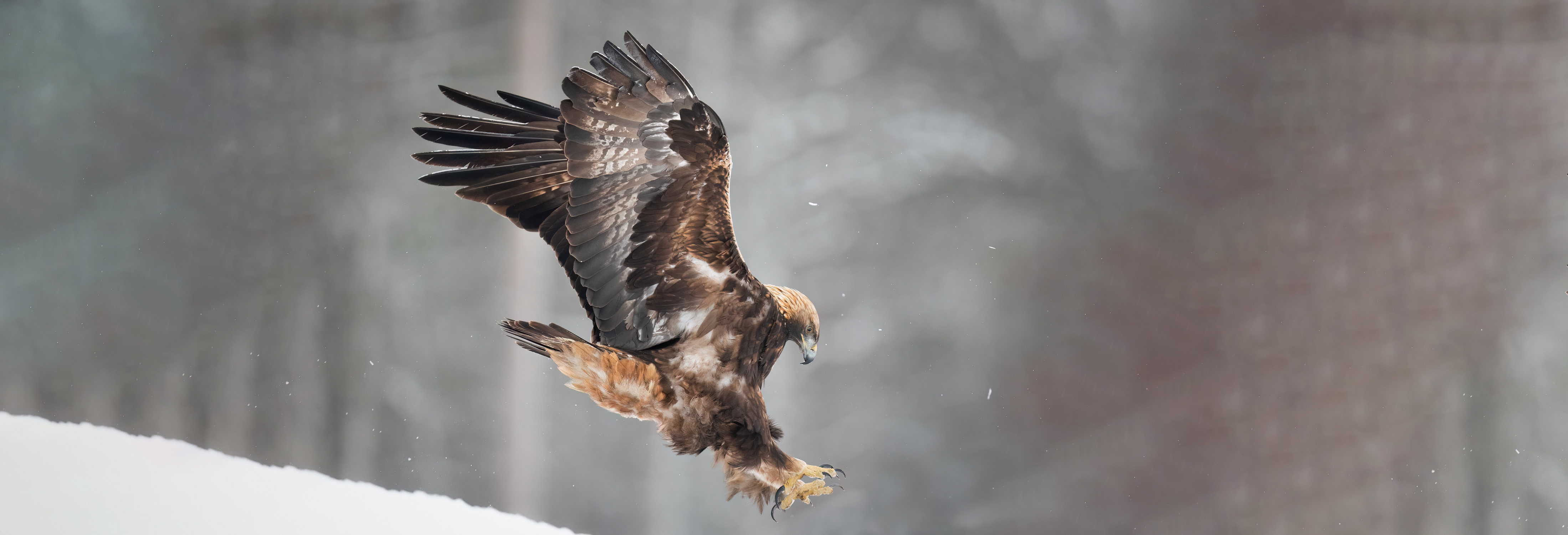
AI for Eagles

AI for Pelicans
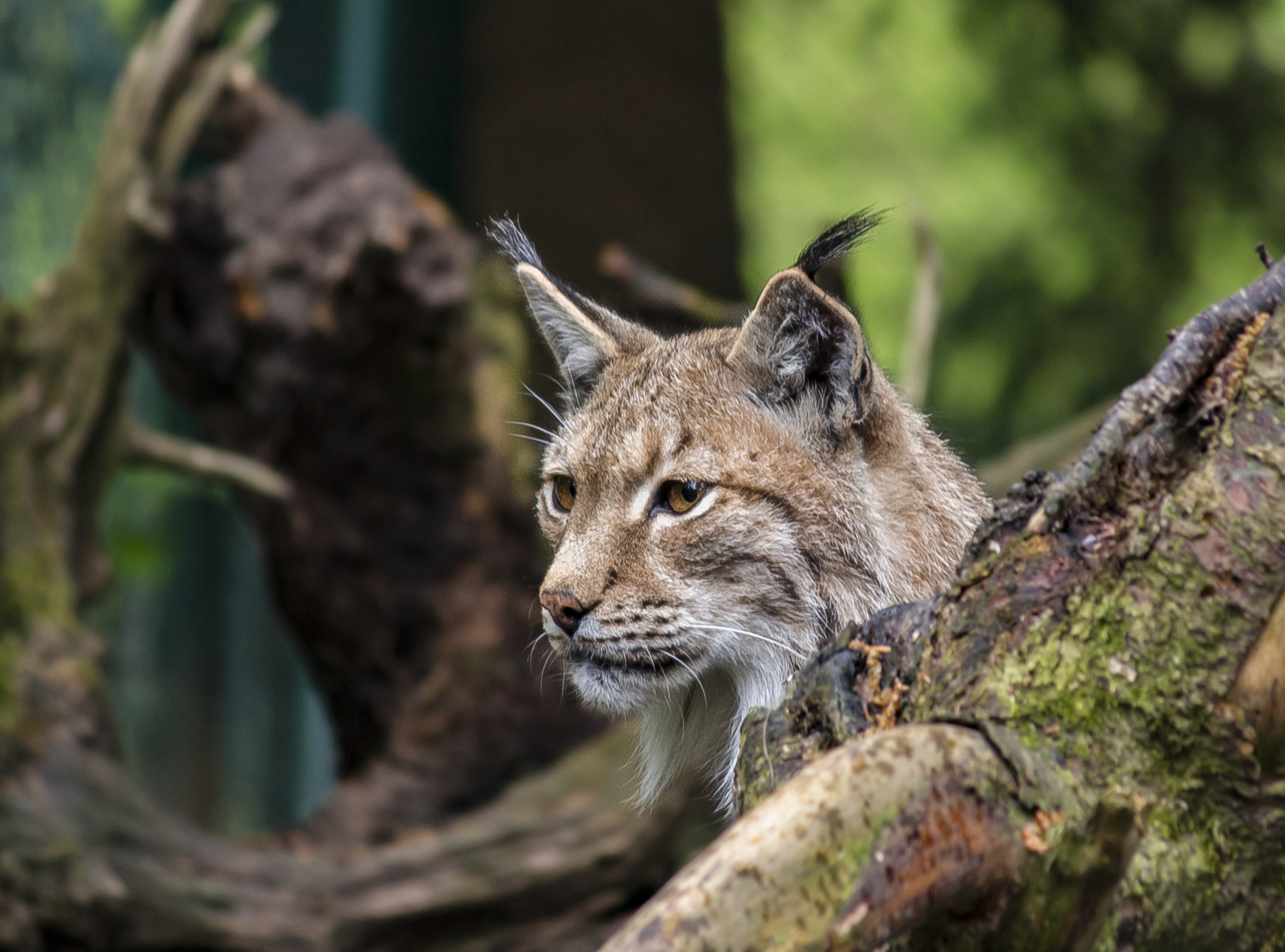
AI for European Wildlife
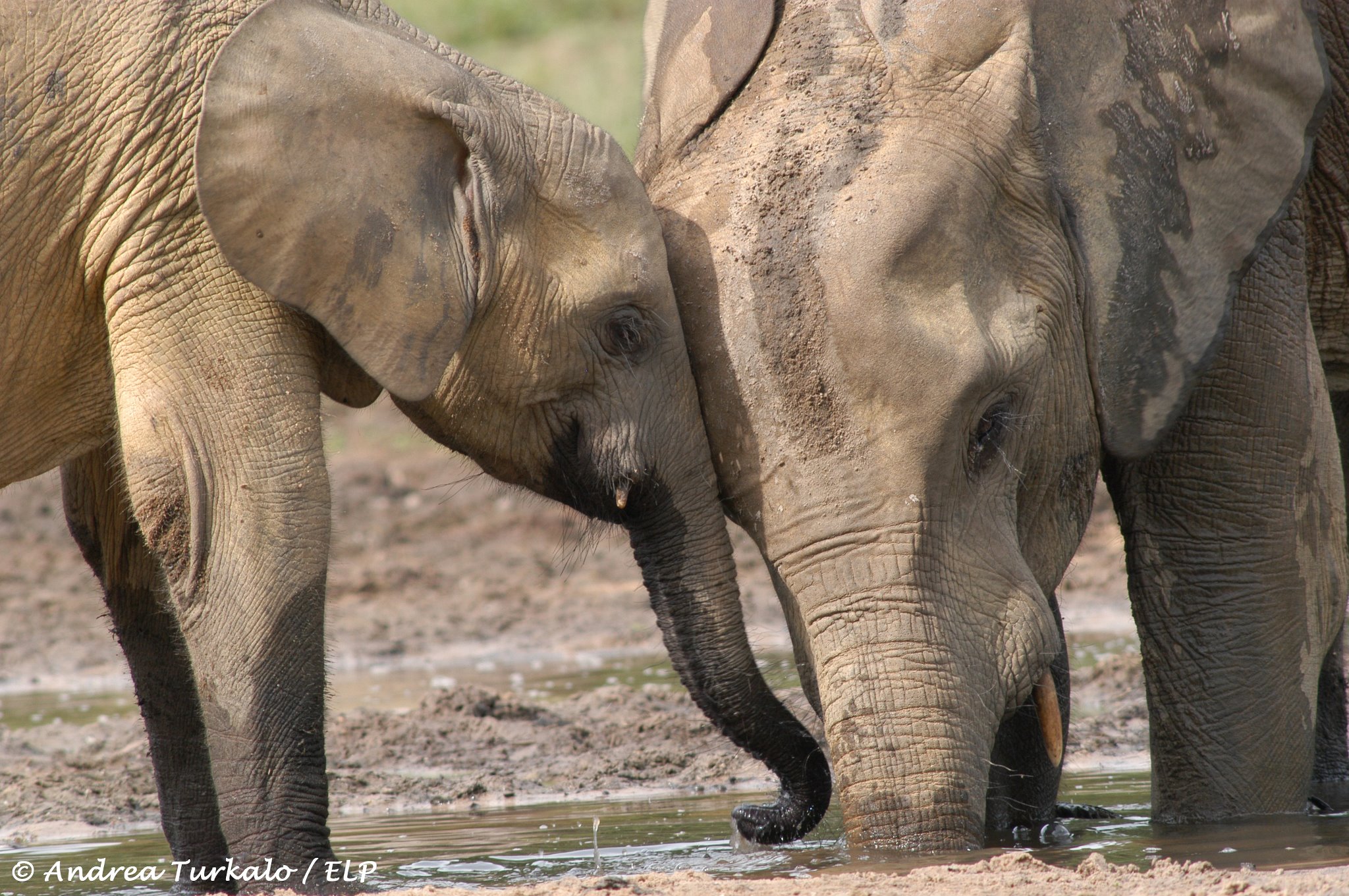
AI for Forest Elephants
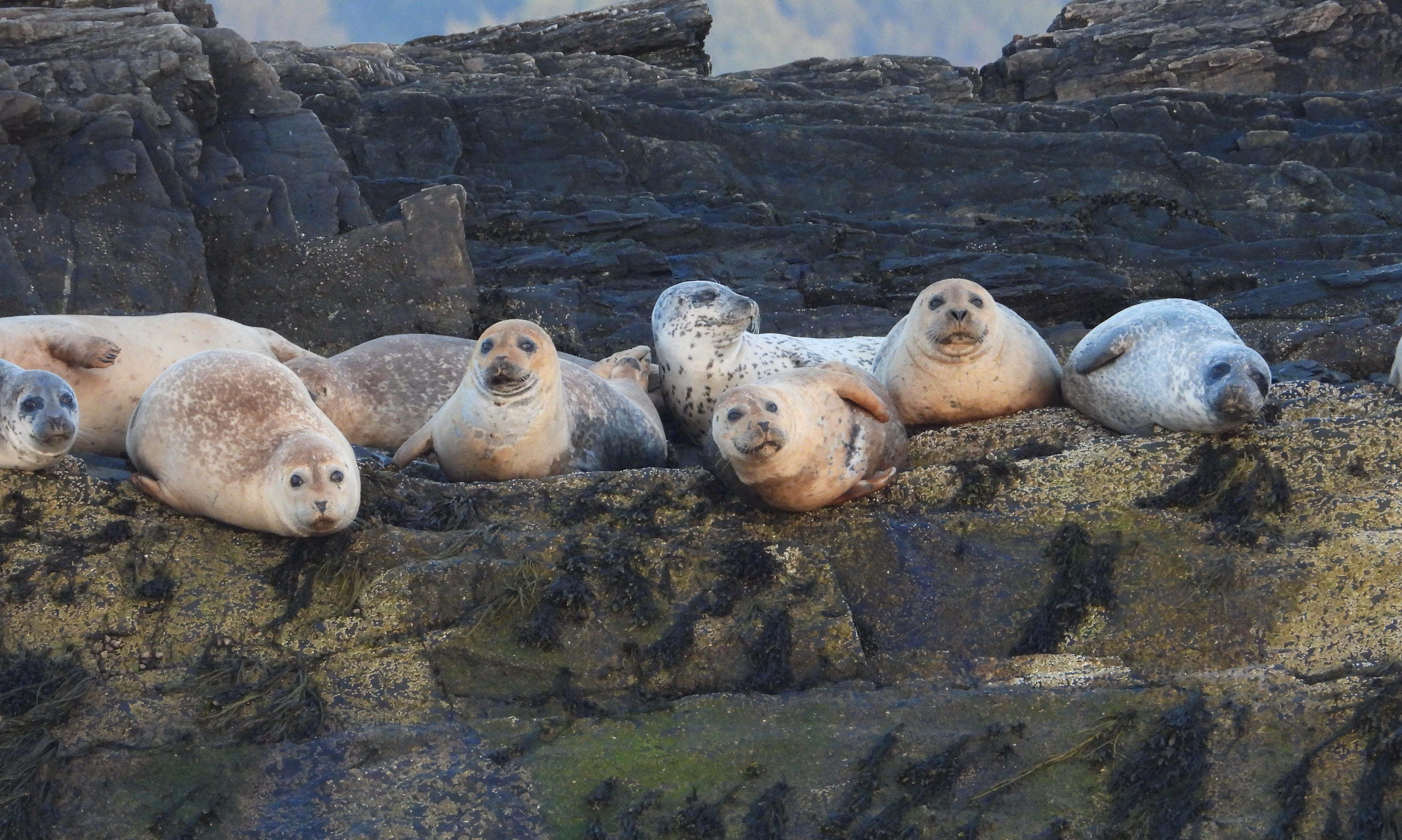
AI for Seals Challenge

AI for Wildlife Challenge 3
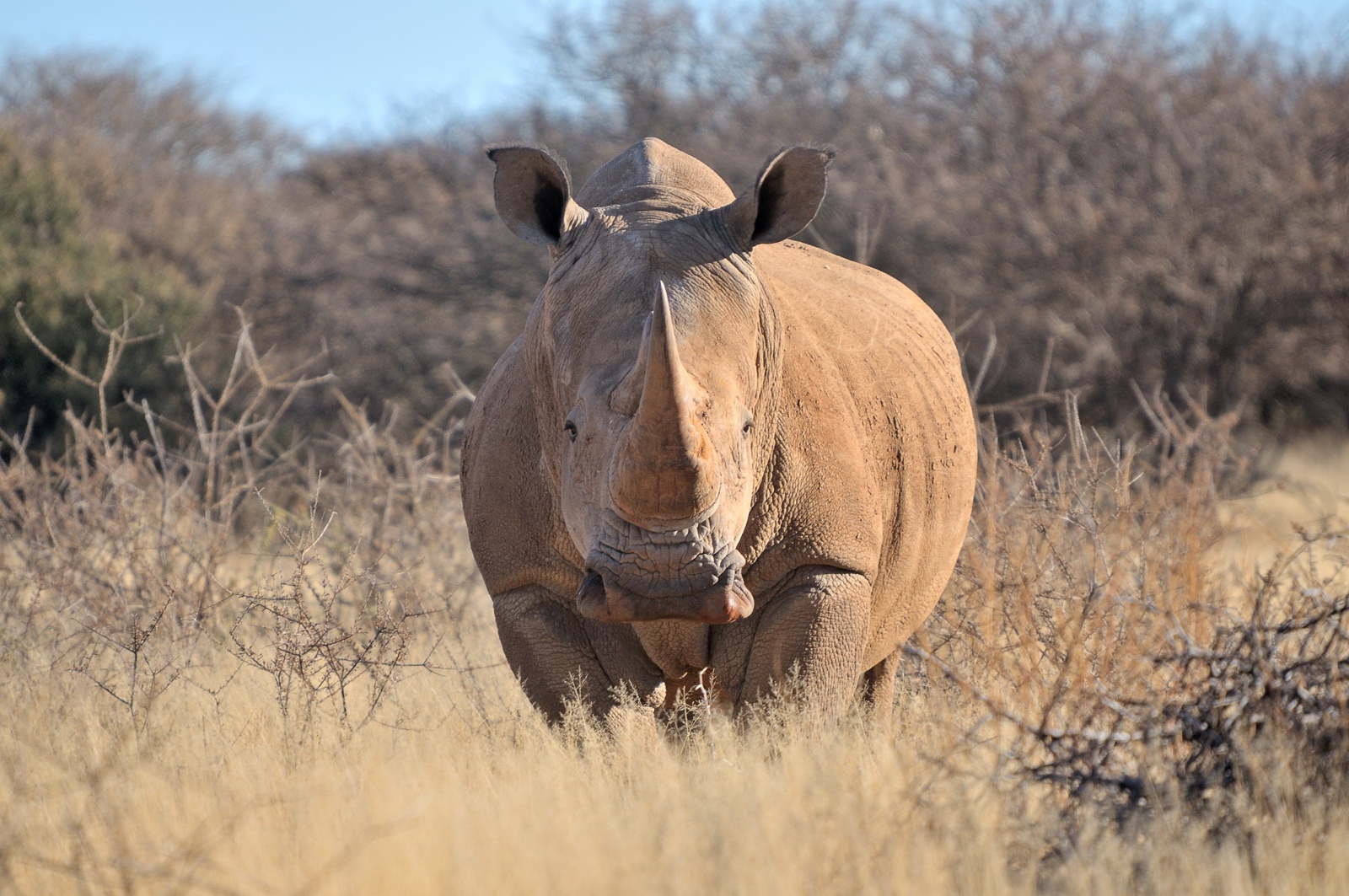
AI for Wildlife Challenge 2
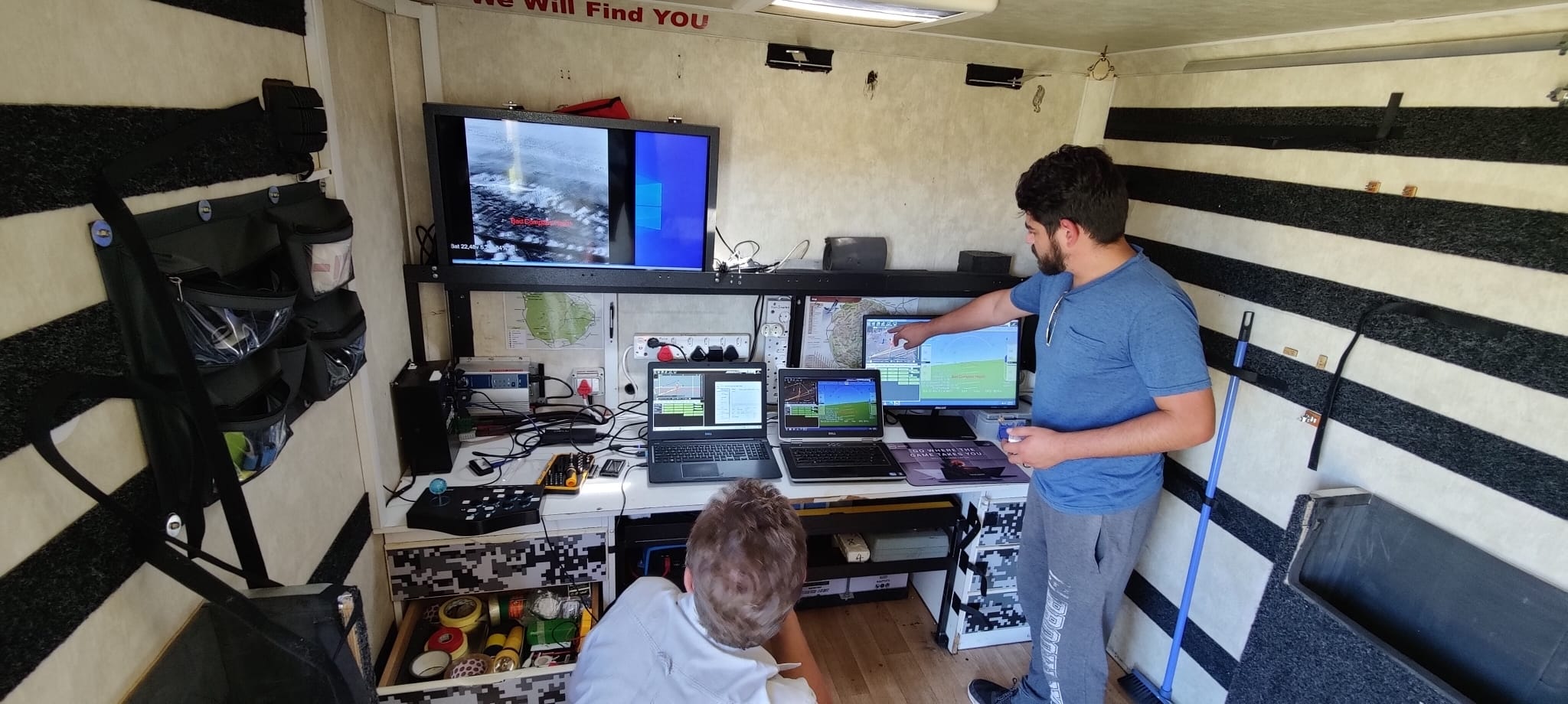
AI for Wildlife Challenge 1
We get some machine learning work done
- Detect poachers on thermal vision on edge of a fixed wing drone
- Do a wildlife sensus using computer vision models on 4k low light RGB aerial footage
- Find patterns in poacher trace reports by using semi-supervised learning
- Apply edge ML poacher detection on battery powered camera traps
- Use audio perception models to identify elephants by their distinctive sounds
- Develop prediction model for elephant migration based on collar GPS data
- Automated operation of big game gates near wildlife reserves
From our YouTube channel
From our Publications
Become a certified AI for Wildlife Engineer
Individual engineers who collaborate in the AI for Wildlife Challenges are trained in edge computing, computer vision, model pruning and other skills essential for the applied AI projects.
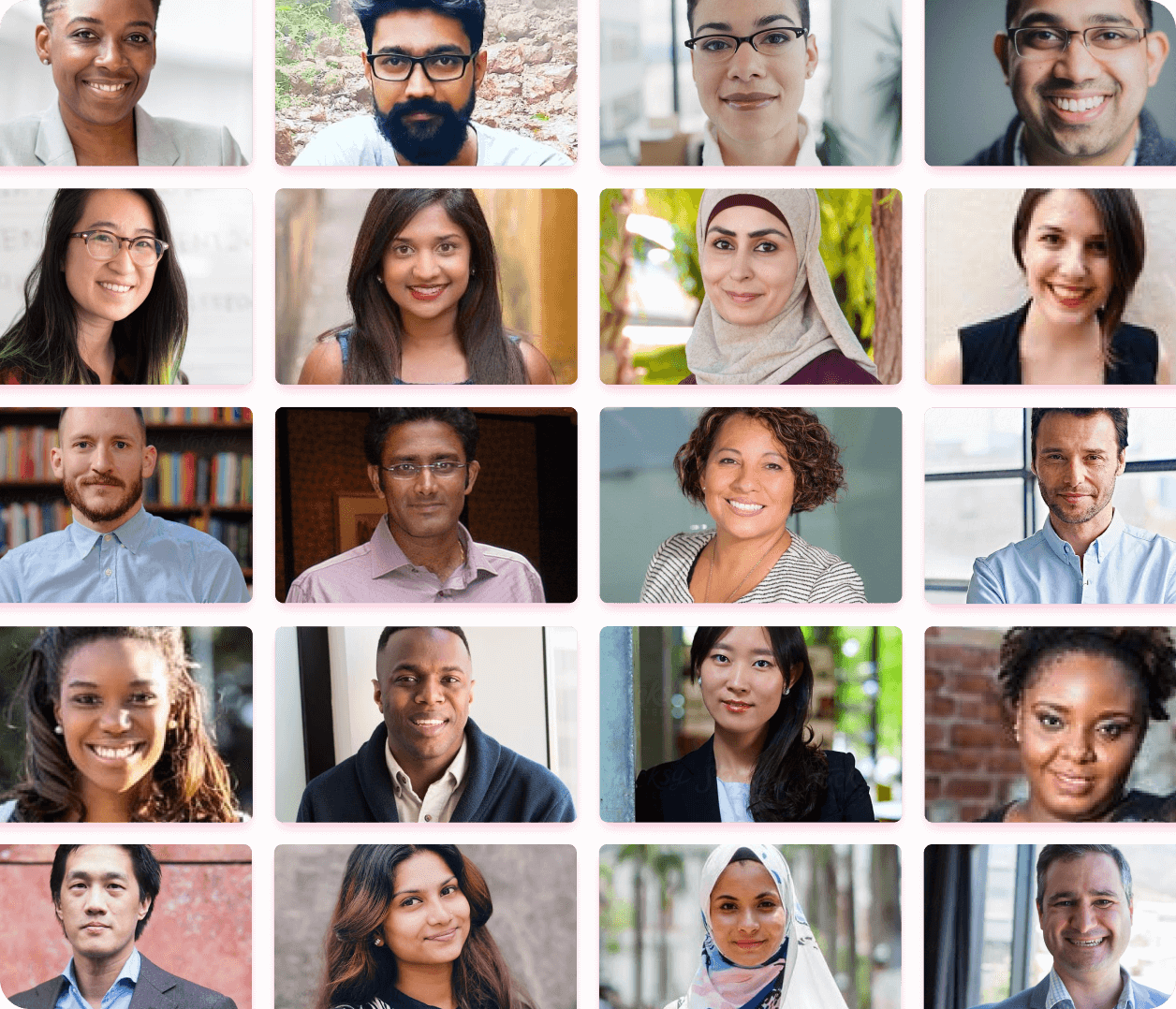
Sign-up at the platform for free and fill your profile. Pick your interests and join corresponding communities. Create a skill tree you’ll be developing with every AI for Good activity.
Start my journey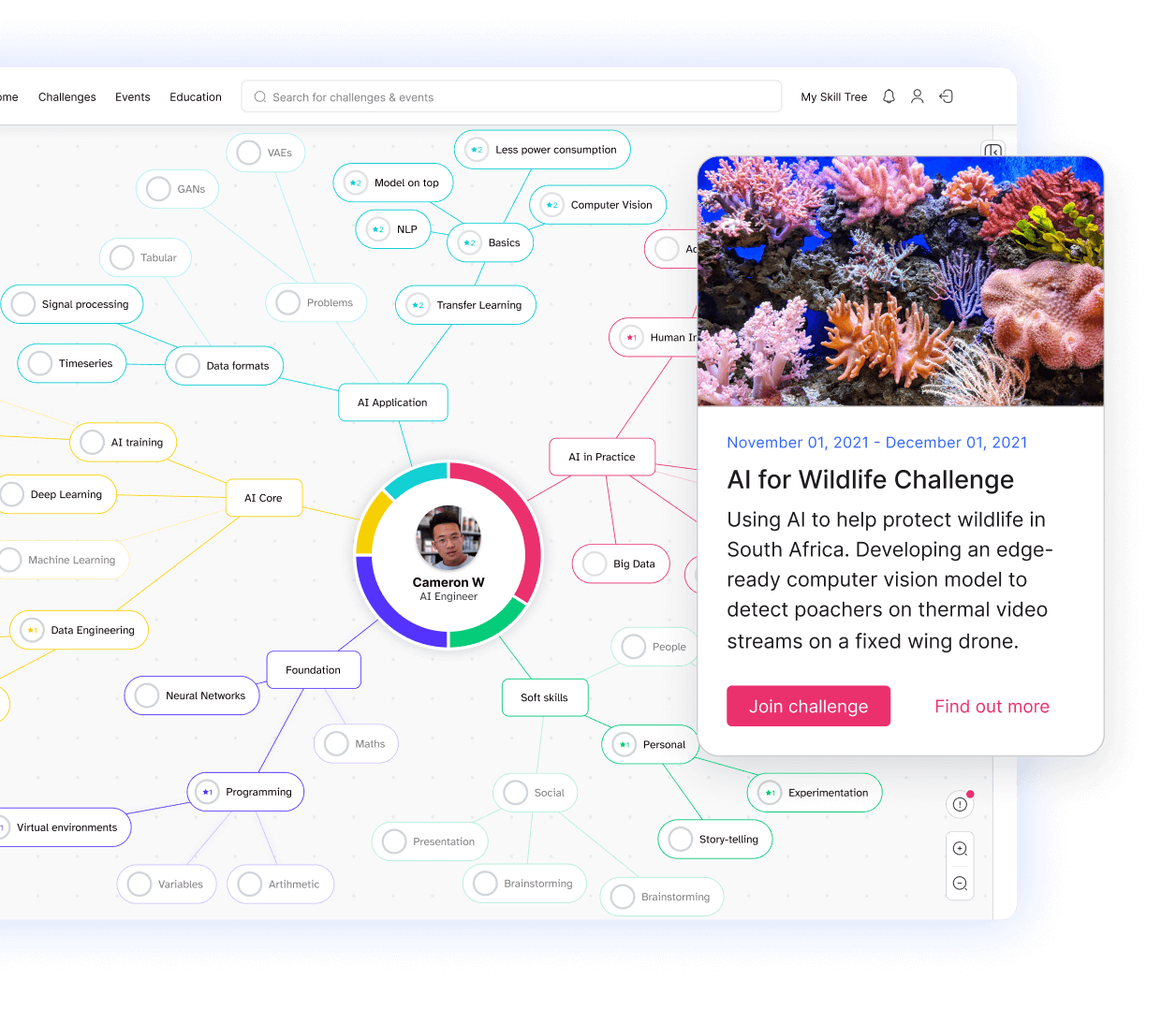
Learn how to apply your acquired AI knowledge in the real world. Join AI for Good challenges and collaborate in teams all over the globe.
Upgrade my skills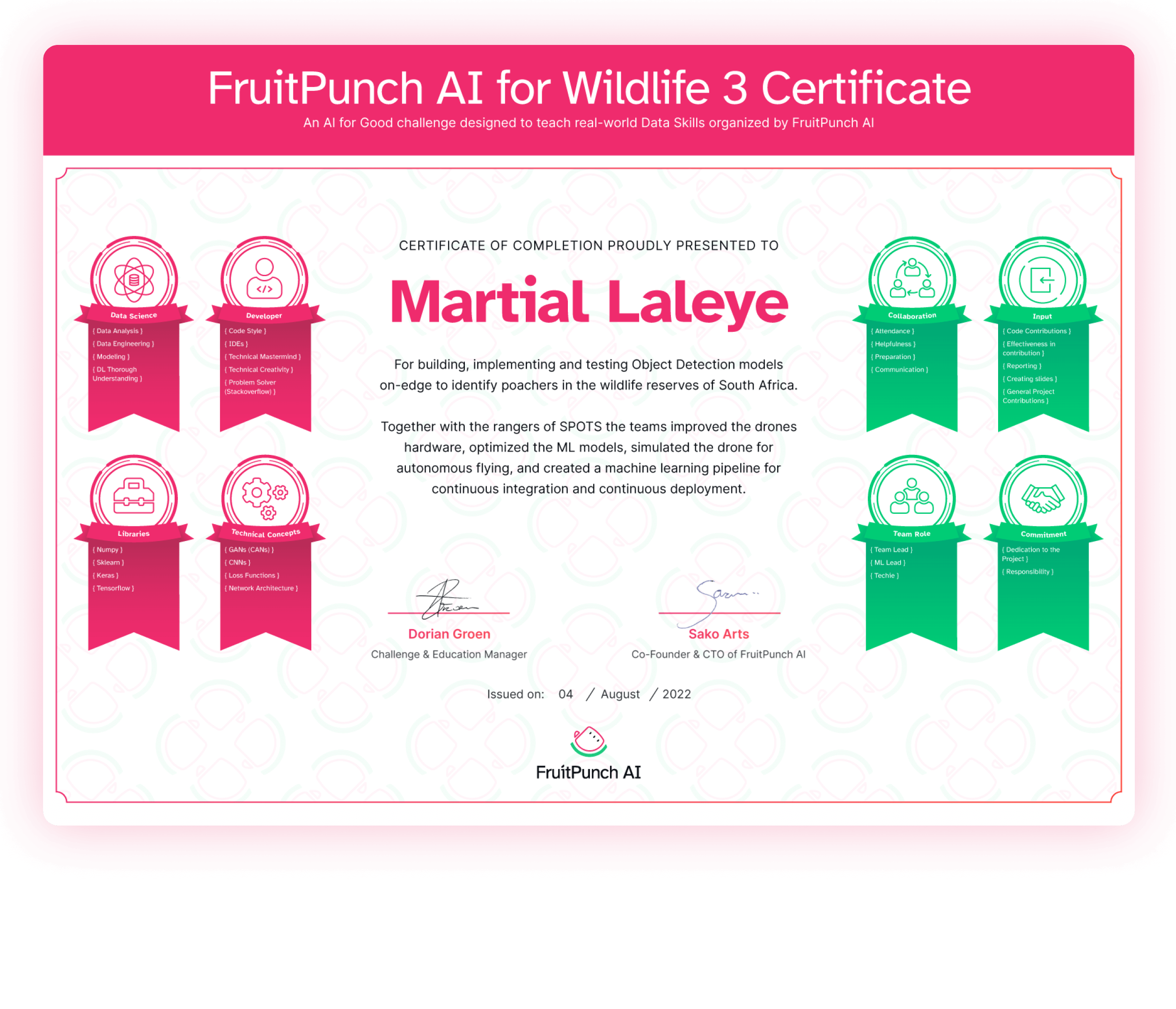
Every activity adds to your development. You’ll be accredited with badges for specific hard and soft skills and certifications after an accomplished challenge.
Build my skill treeBecome a certified AI for Wildlife Engineer
Individual engineers who collaborate in the AI for Wildlife Challenges are trained in edge computing, computer vision, model pruning and other skills essential for the applied AI projects.
Join the community

Participate in a challenge
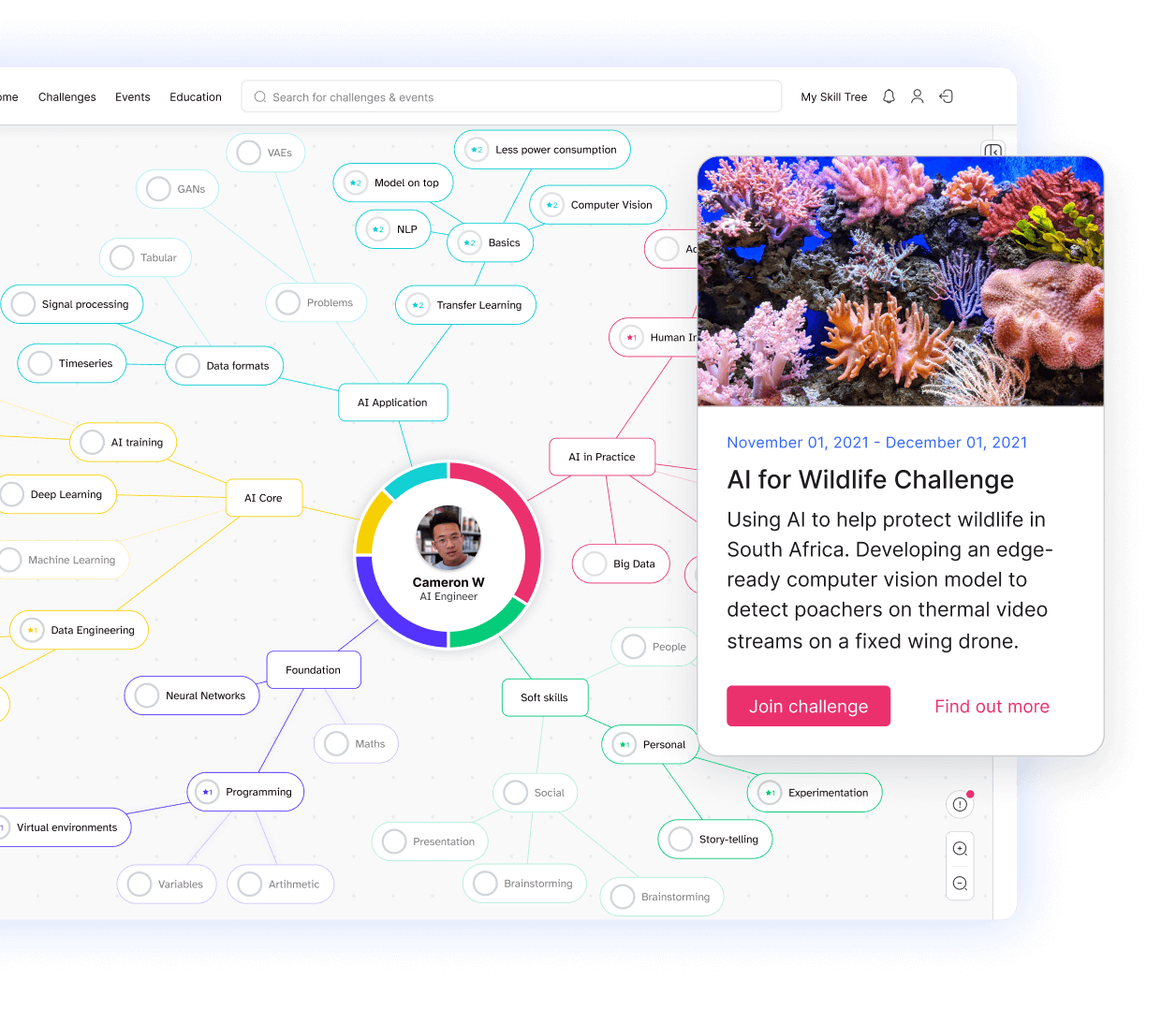
Get certified

Challenge Partners
Impact organizations and experts applying the results of the AI for Wildlife Challenges. Do you have a wildlife conservation problem that can be solved with AI? Submit your challenge
Contributing Partners
Use their teams, expertise and resources to advance AI for Wildlife Lab & Challenges. Can your experts or technology contribute to solving an AI for Wildlife Challenge? Partner up! 👇
Community Partners
Use their reach to spread the AI for Good news, advocate for AI for Good Lab & Challenges. Do you have a network we could reach out to with AI for Wildlife topics? Connect on Linkedin, Twitter or drop us a note 👇